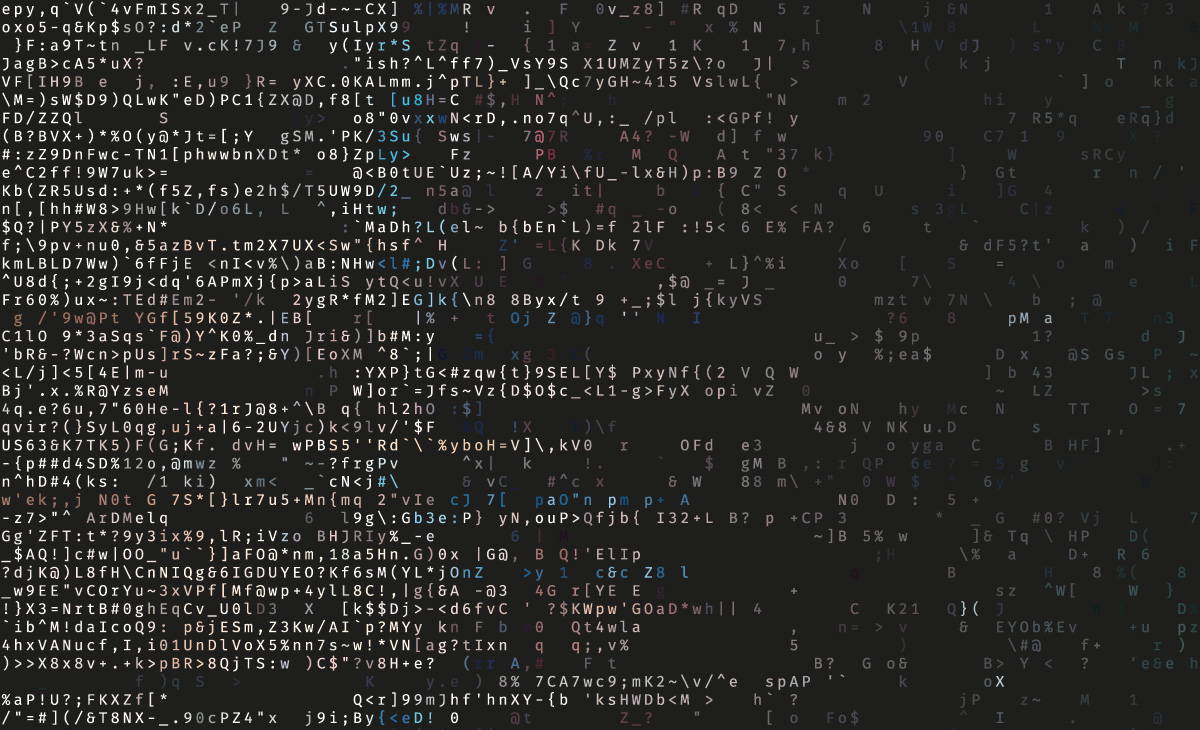
It seems like every couple of weeks we’re treated to some disturbing new story in the press about an institution being rocked by the actions of a rogue employee: a once-trusted insider who then collaborates with foreign spies, or intentionally/unintentionally leaks secrets to unauthorized sources, or perpetrates shocking acts of workplace violence.
Indeed, the prevalence of insider threats, and the devastation they can bring, is one of the reasons why I advocate so strongly for Whole Person threat detection. Operationalized by powerful analytics software, this Whole Person methodology uses social, behavioral and technological indicators to identify problematic employees before they do something harmful.
The challenge, though, is that potential risk indicators are themselves very complex things.
Rather than being generic and interchangeable, each indicator has a certain weight or value that, when determined, can give a security analyst an idea of whether an employee might become an insider threat—and even what sort of insider threat. So, for example, signs of disgruntlement (say, in social media posts or performance reviews) indicate a greater potential for future data theft or sabotage, but they don’t suggest a greater likelihood of becoming a phishing victim.
What’s more, these indicator values—as useful as they are—are not fixed in stone. In fact, the risk values can be reduced through protective factors, i.e., active intervention by the organization getting “left of harm” by helping a troubled individual find an offramp from the critical pathway. This positive intervention might, for example, take the form of the institution providing financial counseling and other resources to debt-burdened employees, thus helping reduce the potential temptation to do something illegal.
Another way the risk values of social, behavioral and technological indicators can change is simply through decay, due to the passage of time. This occurs when, at some point after the first reporting of a risk indicator, the analyst no longer considers it relevant to the insider threat assessment.
The concept of decay is useful to a Whole Person approach to countering insider threats, but until now, the phenomenon has been given short shrift in the professional literature.1 So, to fill in the gaps, let’s begin a foray into the topic of risk decay of potential risk indicators, as well as raise questions for future study.
Now, whether it’s an almost-ripe banana going black after a week out on the kitchen counter or the way our Wi-Fi signal gets weaker the farther we move our wireless device from the home router, decay (biological and technical) is a pretty familiar concept to most people. In a similar vein, the value or weight of a potential risk indicator can attenuate over time (even without the organization’s leadership playing an active mitigation role).
The rate of decay varies across indicators, however. (Why should we expect anything to be simple at this point!) This is because different indicators are judged by expert analysts to have different decay rates.
To illustrate, let’s take the case of Bob, who just returned to work from a vacation—right after his organization required everyone to change their passwords. Bob might experience several failed computer logins, which would normally generate a technical risk indicator. But after a couple months of successful logins, these authentication failures would not be considered problematic. So, this is a case of a relatively high decay rate of a potential risk indicator.
By contrast, Arnold has repeatedly ended up in meetings with HR because of his high-handed and abusive behavior towards co-workers. An analyst would likely judge Arnold to have a character flaw or personal trait that’s not expected to change much over time.2 In such a case, the potential risk indicator would have a very slow decay rate.
Now, as important as risk indicator decay is for fine-tuning insider threat calculations, estimating various rates of decay can be a very labor-intensive process. Thus, an organization might be tempted to defer consideration of potential risk indicator decay and treat all indicators as static over time. This is especially true for counter-insider threat programs with very limited resources.
Treating risk factors as static also services a conservative, overly cautious assessor who never wants to be blamed for letting a bad actor slip through cracks. After all, better to be safe than sorry and treat every indicator as eternally relevant, right?
Not necessarily. There’s a clear downside to treating indicators as static. First, you increase the chance of false positives that waste resources while you chase phantom threats. Second, you also run the risk of alienating employees who have been erroneously targeted—thus creating the very insider threat you are trying to eliminate.
When considering the pros and cons of addressing risk indicator decay, I believe there’s good reason to include it in our threat assessments. We can look at related research on the longevity of grudges, which informs us that grudge-holders who are upset about a particular incident (for example, feeling that a company cheated them in some way) can remain bitter about it for decades.3 Thus, we know that feelings of betrayal—a key predictor of the desire for revenge—decay nonlinearly over time.4
Indeed, exponential decay is a common mechanism. This means that the amount of decrease in a variable, from one time to the next, is proportional to the original value of the variable. So, we can specify a mathematical function that tells us the value of the variable at any point in time, based on its original value and an assumed decay constant. We can also calculate the half-life of the variable (the time it takes to decrease to a value that's half of the original amount), and we can specify a timespan over which the value becomes negligibly small.
Several colleagues and I have conducted studies into the decay parameters of potential risk indicators. First, we conducted expert knowledge elicitation studies to firm up the definitions of risk indicators and get estimates of their strengths (e.g., analyst judgments of the likelihood that a given potential risk indicator indicates a possible insider threat). Then we got their judgments about the rate of change of this risk value over time.
In an initial study,5 we obtained expert estimates of potential risk indicator strength at different points on a timeline and found varying rates of decay for different types of indicators.6 Those connected to personality traits showed very little decay as compared to technological indicators (like authentication errors). This checks out since personality traits are themselves very stable over time.
Thus, we should find decay rates near zero for personal predispositions, but relatively high decay rates for technical indicators—and generally, we do! However, in subsequent studies, I and colleagues at Cogility found numerous exceptions:
- Personal predispositions like psychopathy and narcissism have little or no decay, but so does the technical precursor, Introduction of Malicious Code. Egregious cyber acts are not soon forgotten, unlike lesser cyber events like Printing to Anomalous Location.
- Some behavioral precursors (e.g., Associating with Extremist Groups) have low or no decay, but others (e.g., Attendance Issues) have a moderate decay with a half-life of about two months and virtually ignored after one year.
In summary, potential risk indicators for an insider threat are very complex things to calculate, so the temptation is to simply err on the side of caution and treat them as having static values. This neglect of decay is problematic because it can lead to rather expensive wild goose chases by an organization’s security analysts and can also cause the problem you are trying to avoid: aggrieved employees who might seek revenge in the future.
I believe indicator decay is a worthy topic of study in countering insider threats within a Whole Person framework. And yet, our understanding of the subject is still in its early stages. Based on my own research and the nuances revealed, I think it's best to estimate decay parameters on a more granular level instead of using role-type categories like predispositions, technical or behavioral factors, as was described in my earlier work.
Indeed, a deeper exploration of this topic is needed to further advance the models. Here are some topics that we in the security community should be exploring next:
- In my research to obtain expert judgments of potential risk indicator decay rates, I observed that analysts were reluctant to assign very high decay rates (half-life = 1 week)—even for technical indicators. This may reflect a desire to avoid overlooking issues of concern. More than half of the technical indicators were assigned a decay rate with a half-life of one or two months, but 9% were considered to not decay at all. By contrast, 69% of personal predispositions were assigned zero decay rates. While these findings confirm the hypothesis that indicators have varying decay rates, more careful study is needed to better characterize the underlying factors.
- My 2022 study with Justin Purl also took an initial, limited look at the effects of intervening potential risk indicators that occur after an initial occurrence. More detailed study is needed, especially for cases involving the repeated occurrence of a potential risk indicator that had undergone some decay.
- In the above discussion, I referred to factors other than time that can decrease the impact of an indicator, viz., mitigating protective factors. Conditions that diminish the impact of a potential risk indicators (besides decay) represent another area for further study.
References
- Greitzer, F. L. & Purl, J. (2022). The dynamic nature of insider threat indicators. Springer Nature Computer Science, 3(102); Greitzer, F. L., Kliner, R. A., & Chan, S. (2022). Temporal effects of contributing factors in insider risk assessment: Insider threat indicator decay characteristics. WRIT Workshop, Austin, TX, December 5, 2022.
- Cobb-Clark, D. A. & Schurer, S. (2012). The stability of big-five personality traits. Economics Letters, 115(1), 11-15.
- Hunt, H. K., Hunt, H. D., & Hunt, T. C. (1988). Consumer grudge holding. Journal of Customer Satisfaction, Dissatisfaction, and Complaining Behavior, 1, 116-118.
- Gregoire, Y., Tripp, T. M., & Legoux, R. (2009). When customer love turns into laying hate: The effects of relationship strength and time on customer revenge and avoidance. Journal of Marketing, 73(6), 18-32; Tripp, T, M., & Gregoire, Y. (2011). When unhappy customers strike back on the Internet. MIT Sloan Management Review, Reprint 52303, pp.1-8.
- Greitzer, F. L. & Purl, J. (2022).
- Ibid.