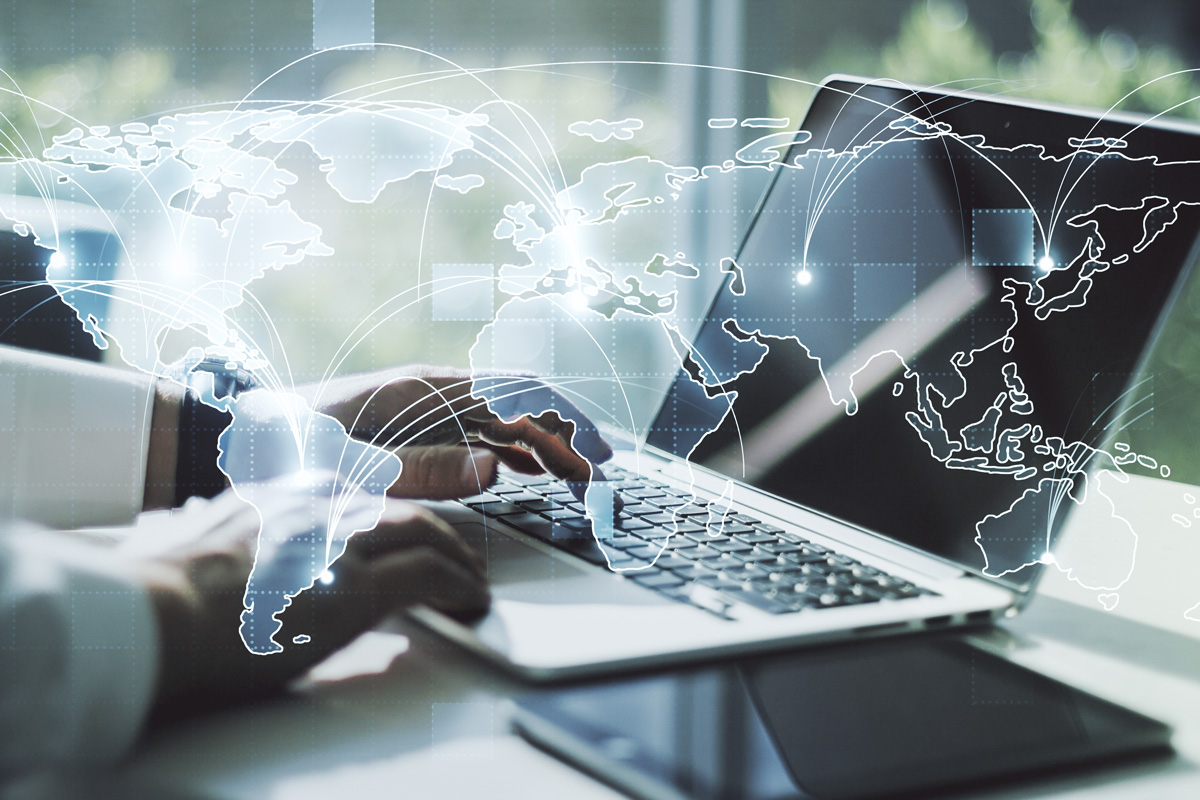
In March 2024, Belgium and the United States co-hosted the 2nd International Symposium on Mitigating Insider Threats. Experts from around the world discussed current and emerging issues relevant to nuclear security and insider threat mitigation. Frank Greitzer presented a talk on his research in developing the SOFIT insider threat indicator knowledge base.
To advance the core tenants of IAEA INFCIRC/908 and Revision 1, the Advancing INFCIRC/908 International Working Group is producing a webinar series that revisits select content from the March 2024 Symposium, including Frank’s April 2025 webinar that reprised and updated his Symposium presentation:
“Exploring Predictive Analytic Threat Assessment Models for Proactive Insider Threat Mitigation”
Based on two decades of research, the presentation discussed technical challenges and recent insights in developing and testing behavioral science-based models for proactive insider threat mitigation and featured the updated SOFIT insider threat indicator knowledge base. The presentation may be viewed at the INFCIRC website and here.
A Q&A session followed the presentation, but since there was not enough time to address all the questions that were contributed by attendees, this blog provides a more complete set of answers.
- Within the SOFIT model where do most organizations get it wrong?
The SOFIT framework encourages organizations to consider behavioral factors that support a more proactive approach that aims to identify at-risk individuals and apply mitigation strategies to help them find an “offramp” from the critical pathway. However, if the insider risk analysts focus on individual PRIs rather than combinations, this can undermine the whole-person/proactive mitigation strategy. Many individual PRIs are not sufficient, by themselves, to trigger an insider risk alert, but when considered together with other PRIs, this pattern may yield an actionable insider risk assessment. - How do the models account for the potential for human error or bias in the data collection and analysis process?
As described in a 2022 INSA white paper on bias, “Bias is a pattern of decision-making that favors one group, person, or thing over another, while unfairly discriminating against the remainder of the choices.” As described in the white paper, human sources of bias (“cognitive biases”) include the availability bias (e.g., bias toward familiarity), confirmation bias (e.g, interpreting information in ways that support existing beliefs), and anchoring bias (e.g., initial evidence may be weighted more heavily than later information), among others. Ways that decision makers overcome cognitive biases include structured decision making strategies and effective anonymization of data. For insider threat programs, a multidisciplinary insider risk management team comprising individuals with diverse backgrounds, professional experience, and representing different disciplines will help to reduce various biases.The models I have studied are built upon expert judgments, and as such, biases that are present in expert judgments can be reflected in the models. One way to reduce the impact of individual biases on decision support tools is to aggregate the judgments of many experts, across several disciplines, rather than basing the model on a single or just a few experts. When we conduct expert knowledge elicitation exercises to help inform our models, we look for experts with a range of backgrounds and disciplines including security, cybersecurity, human resources, behavioral sciences, legal, etc.
- What position in a company is best suited to detecting internal threats?
“It takes a village.” The insider risk management team should be staffed by both technical/IT/cyber specialists and behavioral science professionals so that a wide range of concerning behaviors may be recognized. - Are these AI supported tools and if so what tools have been developed to combat biases in AI programming?
The models I described represent a range of sophistication. The simplest models (“Counting Model” and “Sum-of-Risk Model”) are not AI tools. The Cogynt model developed by Cogility Software is an AI-based model. I also mentioned other types of models, such as those that use machine learning to support decision-making. Most of these more sophisticated models are developed to conform to expert assessments: any models that reflect expert judgments can be subject to human biases. As described in the answer to Question #2, there are approaches designed to minimize the contribution of human bias.AI programming may introduce new biases as well. If a model is trained on a narrow range of data, it may properly reflect expert judgments for that type of data, but it may be inadequate to address new situations. In other words, if you develop a model to identify at-risk individuals for insider threats of exfiltration or espionage, your model may perform adequately for this context, but it might perform poorly in identifying possible perpetrators of fraud. I can provide empirical evidence for this sort of challenge in the context of phishing susceptibility: In a study published in 2021, we found that technical data (cyber host/network data) was completely ineffective in identifying individuals who were more likely to fall for phishing emails—only certain behavioral factors were related to phishing susceptibility. [Greitzer, FL, W Li, KB Laskey, J Lee, & J Purl. (2021). Experimental Investigation of technical and human factors related to phishing susceptibility. ACM Transactions on Social Computing, 4(2), Article No. 8, June 2021, pp.1-48. https://doi.org/10.1145/3461672]
- What is the adaptability of ontology to cultural and both interorganizational and intraorganizational variability?
The SOFIT knowledge base was developed based on expert judgments of primarily U.S. insider risk analysts and researchers. We haven’t conducted any multicultural knowledge engineering studies or made any attempt to determine the extent of cultural differences. I would expect that the technical indicators are relatively robust with respect to inter/intra organizational differences, or even cultural differences. However, cultural factors are likely to produce differences in the calibration weights of risk indicators. - What are the advantages of behavioral science based methods for insider threat mitigation?
This is a major theme of my presentation and I would refer you to review the video and the slides that I discussed. Also perhaps look a this paper: Greitzer, FL. (2019). Insider Threat: It’s the HUMAN, Stupid! Proceedings of the Northwest Cybersecurity Symposium, April 8-10, 2019. Article No. 4, pgs 1-8. ACM ISBN 978-1-4503-6614-4/19/04. https://doi.org/10.1145/3332448.3332458 - How effective have certain psychological profiling methods been at identifying insider threats early enough to prevent a crime?
I’m not aware of published case studies that demonstrate utility of insider risk mitigation programs in preventing real-world insider exploits, whether encompassing behavioral indicators, technical indicators, or both. There may be data or reports on this topic, but I have no knowledge of any publicly available reports or any peer-reviewed scientific papers. - Was the model tested on past real cases (attempts or acts)? If so, what scoring would have been applied?
The statistics and metrics presented in recent conferences (and this webinar) reflect testing of models based on simulated data, with a select number of cases that were loosely modeled after real cases. Because specific details of insider incidents are typically unavailable (except for accounts described in public sources), it was not possible to exactly duplicate these cases. The tests of the models consisted of determining if the risk scores produced by the models were consistent with “triage recommendations” of expert judgments of the cases. In past research, this was assessed based on the correlation between the expert judgments and the model outputs. More recently, we have applied other metrics that derive from signal detection theory and data mining approaches. - How can we identify someone suspicious within the organization? (because she / he behaves [and] looks like normal as the other[s])?
This is a great question that speaks to the crux of the problem in identifying insider threats. In fact, in a panel session called “Artificial Intelligence, Machine Learning, and the Cyber-Inside Nexus” at the 2024 International Symposium on Insider Threat Mitigation in Brussels, Belgium, I noted that “most of the time the malicious insider behaves and looks much the same as innocent individuals.” I’m reminded of the “Policeman’s Song” from the Gilbert & Sullivan opera, Pirates of Penzance (1879):“When a felon’s not engaged in his employment
Or maturing his felonious little plans,
His capacity for innocent enjoyment
Is just as great as any honest man’s.”Just as the policeman (and the person who asked this question) lament, insider threats spend most of their time exhibiting behaviors that are indistinguishable from normal staff.
This suggests that the popular anomaly detection approach in cybersecurity is the wrong paradigm for insider threat detection. Anomaly detection approaches assume that normal and malicious behaviors are separable: i.e., malicious actions are outliers when compared with normal activity. This is the basis for using AI solutions based on machine learning models for anomaly detection. While these approaches work well in some domains, they do not readily apply to insider threats. See for example, the study described here: Liu, A., Martin, C., Hetherington, T., & Matzner, S. (2006, March). AI Lessons Learned from Experiments in Insider Threat Detection. In AAAI Spring Symposium: What Went Wrong and Why: Lessons from AI Research and Applications (pp. 49-55). https://cdn.aaai.org/Symposia/Spring/2006/SS-06-08/SS06-08-013.pdf
This is one of the reasons why I prefer to explore AI approaches that more explicitly model the insider risk analysts decision process, rather than the “black box” anomaly detection methods employed in machine learning models.
- How effective are predictive analytics-based threat assessment models in identifying and mitigating insider threats in critical infrastructure systems?
Interesting question: Unfortunately, I do not have any information about this... - Can behavioral analytics combined with machine learning improve the accuracy of insider threat prediction in enterprise environments?
I believe that behavioral analytics, combined with any quantitative modeling approach, will improve the accuracy of insider threat predictions over a baseline that doesn’t include behavioral data. Machine learning methods may be applied to help fine-tune the PRI “weights” in a predictive model such as Cogynt. However, one limitation of machine learning approaches is that the scope of application of the machine learning model is constrained to the range of behavior covered in the training data, so the model will only be as good as the scope and breadth of the data to which these methos are applied. In other words, one is always “fighting the last war rather than the next one.” For this reason, I have advocated the use of expert knowledge elicitation studies that include synthetic/hypothetical cases in addition to cases that reflect the organization’s historical data. - What metrics best evaluate the performance of predictive analytic models in mitigating insider threats proactively?
There’s an INSA white paper on the topic of Measuring the Effectiveness of Insider Threat Programs. Measures of effectiveness (MOEs) for operational programs include number of cases generated per week, average time required to detect a threat, average time to resolve a case, etc. These types of MOEs represent the operational capacity or “throughput” of the insider risk assessment program. They were developed for and apply well to “reactive” threat assessment environments.They may be applied in proactive environments, but the success criteria for proactive programs are somewhat different. I recently wrote a blog about measuring the performance of insider risk mitigation models or programs. Titled “Keeping Those Elephants Away: A Force Multiplier for Insider Threat Analysis,” this essay describes the story of a man who claps his hands every ten seconds. When asked why, he says: “I’m clapping to scare away the elephants.” When it’s pointed out to him that there are no elephants around, he says, “See? It works!” (This fable is described in the book, The Situation is Hopeless, But Not Serious [The Pursuit of Unhappiness], by Paul Watzlawick (1983). Norton, Chapter 6, p. 53.)
This example of non-scientific or “magical” thinking emphasizes the difficulty in assessing the impact of a proactive insider risk mitigation program: It’s not always easy or obvious how to count the absence of an effect!
Typical MOEs do not readily apply to proactive programs aiming to prevent or mitigate the risk. Appropriate performance measures for proactive programs should not only include the more traditional “throughput” metrics, but also should reflect successful interventions or mitigations that either prevent the threat entirely or reduce the impact.