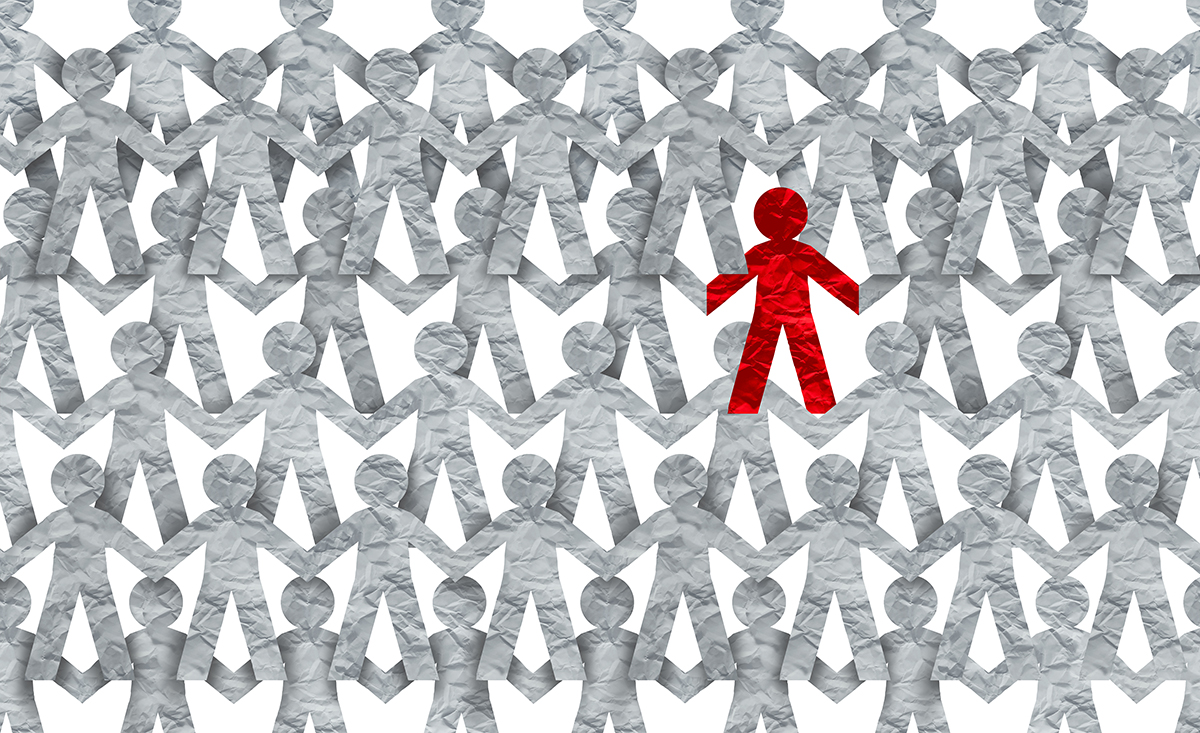
In 1885, Captain Alfred Dreyfuss, a French Jewish artillery officer from Alsace, was convicted of treason for supposedly offering to sell military secrets to the German Empire. For this crime, he was disgraced and sent to Devil’s Island to serve a life sentence in a penal colony.
Now, there was no doubt that someone had been in contact with the Germans. French intelligence had retrieved the memo offering the sale of military secrets.
But the evidence against Dreyfuss was weak. It rested on the fact that he’d visit his family in Alsace (then occupied by the Germans) and his handwriting looked nothing like that of the traitor and, therefore, in the convoluted logic of an “expert” graphologist, it was a so-called “self-forgery.”
Eventually, though, the French public learned that there had been a rush to judgment and a military cover-up. After five years of being on Devil’s Island, Dreyfuss was pardoned and, in 1906, reinstated to the French army. But the real traitor, a debt-ridden major named Ferdinand Walsin Esterhazy, never paid for his crime.
Bias had sabotaged the French army’s hunt for a turncoat. Likewise, bias can cripple any organization’s Insider Risk Management (IRM) program.
What is bias exactly? The Insider Threat Subcommittee of the Intelligence and National Security Alliance (INSA) describes it in a white paper as “a pattern of decision-making that favors one group, person, or thing over another, while unfairly discriminating against the remainder of the choices.”1 To combat potential bias, IRM programs must uniformly and consistently monitor all personnel equally for technical and behavioral patterns of insider risk.
When an analyst’s judgment is skewed by personal characteristics (race, nationality, gender, etc.), or when decisions are based on inaccurate/incomplete data or a flawed model, this can result in misidentifying an innocent employee as a malicious actor or in missing a real threat.
A biased IRM program can also alienate employees who feel unfairly targeted. It can decrease morale, reduce productivity, and lower employee retention. All this can increase insider risk. What’s more, the organization is now exposed to legal action, regulatory scrutiny, and reputational harm.
This is why it is critical to identify and counter the various forms of bias in insider risk programs.
With that in mind, first consider some relevant human biases, especially these cognitive biases2, 3:
- Confirmation Bias—the tendency to search for and interpret—or to disregard or discount—information that shores up one’s prior beliefs.
- Availability Bias—allowing the most available or familiar information (for example, events being discussed in the media) to skew the prioritization of threats.
- Anchoring Bias—using preliminary information as a reference point for interpreting later information, making the decisionmaker less inclined to adjust hypotheses in light of new events.
- Authority Bias—allowing an authority figure to unduly influence decisions.
- Cognitive limitations—taxing the mental capacity of people to the point where they produce errors and omissions that bias decision outcomes.
Cognitive biases influence insider risk analysis in different ways. For example, confirmation or anchoring biases may come into play if they reflect racial disparities in arrest records collected during background checks.
Human biases also arise from cognitive limitations: These can impact the risk assessment triage process when analysts and subject matter experts (SMEs) assign risk values to potential insider risk indicators (PRIs). Errors made by analysts in judging the values of individual PRIs can be multiplied when their combined impacts are assessed.
To counter human biases, one should:
- Adopt hiring practices that promote diversity and inclusion to counteract personal biases.
- Increase the number of SME resources to promote diversity of opinion.
- Provide decision support tools to mitigate the limitations in human memory/information processing.
- Anonymize data to mask individual identities, thus reducing assessments influenced by stereotypes.
Next, let us consider possible technical biases.4 These are associated with the methods and computational models used to calculate risks as well as the application of Artificial Intelligence (AI) to IRM. The following are of particular concern:
- Selection Bias—including certain types of data, while excluding others.
- Model Bias—developers or domain experts may inadvertently code their own biases into a model or rely on explicit assumptions not met in the real-world dataset.
- Biases in use of AI/ML technologies—training AI/ML models on restricted data sets or applying models developed for one domain to a different one.
Technical biases can be subtle, but they are just as dangerous as human biases. For example, the preference for collecting and analyzing technical data (more readily obtained from IT/cybersecurity resources) to the exclusion of behavioral data (from, say, Human Resources), exemplifies Selection Bias5.
Detection tools drawn from the world of cybersecurity (such as anomaly detection techniques) can be a poor fit for detecting malicious insiders because, as noted in the “Policeman’s Song,” in The Pirates of Penzance, bad guys act like good guys most of the time.6 Similarly, applying a model developed to detect one type of insider threat (e.g., fraud) will not be as effective in detecting another insider threat (e.g., workplace violence).
Finally, there is a subtle human tendency to trust AI solutions. With the advancement of generative AI and LLMs, this trend is only growing since interactions with LLMs are increasingly difficult to distinguish from human interactions. As Charles Owen-Jackson (2024)7 notes in Security Intelligence, the anthropomorphizing of AI tools makes us even more disposed to trust AI when making decisions. What’s worse is that generative AI models—while not trained to deceive us—can produce misleading content or so-called “hallucinations.”
A notable example of such a hallucination, cited by The New York Times, is the case of Mata v. Avianca in which Roberto Mata’s lawyer, Steven A. Schwartz, used ChatGPT to help with his legal briefing and ended up citing three cases that had been completely invented by AI. When the hallucination was discovered, Schwartz told the court that he didn’t even realize that an AI tool could spit out such false information.
But it can.
To mitigate these technical biases, one should:
- Use high-quality training data to develop AI/ML models, ensure that the data used comes from independently validated sources, and have humans fact-check the AI/LLM outputs.
- Ensure data is not overly focused on a particular demographic group.
- Make models transparent—enabling internal and external audits of the algorithm, extensive testing, periodic refinement of datasets, and retraining of models.
- Use caution in repurposing analytic models.
The Dreyfuss Affair is a cautionary tale for the insider risk community. Biases—implicit and explicit, human and computer—can undermine our programs. Of course, the remedy is not to “throw the baby out with the bathwater.” Subject matter experts and automated support tools, including AI models, are invaluable tools. But we must be smarter and more transparent in how they’re employed.
So, in summation, we in the insider risk community need to take potential sources of bias very seriously: The Dreyfuss Affair is a cautionary tale that can easily happen to us. But if instead of repeating the mistakes of the past, we open our eyes to biases—old and new, implicit and explicit, human and computer—we can more effectively counter them before they undermine our programs.
1 Intelligence and National Security Alliance. (2020). Human Resources and Insider Threat Mitigation: A Powerful Pairing. INSA Insider Threat Subcommittee White Paper, September 2020. https://www.insaonline.org/docs/default-source/uploadedfiles/2020/01/insa-int-sept252020.pdf
2 Kahneman D, Tversky A (1972). "Subjective probability: A judgment of representativeness" (PDF). Cognitive Psychology. 3 (3): 430–454. doi:10.1016/0010-0285(72)90016-3.
3 Haselton MG, Nettle D, Andrews PW (2005). "The evolution of cognitive bias.". In Buss DM (ed.). The Handbook of Evolutionary Psychology. Hoboken, NJ, US: John Wiley & Sons Inc. pp. 724–746.
4 NIST Special Publication 1270: Towards a Standard for Identifying and Managing Bias in Artificial Intelligence. https://nvlpubs.nist.gov/nistpubs/SpecialPublications/NIST.SP.1270.pdf
5 In my blog on the Whole-Person Approach, I described this bias toward exclusive use of technical data. This selection bias is also illustrated in the “Streetlight Effect” [Mutt & Jeff comic strip, Boston Herald, May 24, 1924, Whiting’s Column: “Tammany Has Learned That This Is No Time for Political Bosses,” Page 2, Column 1, Boston, Massachusetts]
6 William Schwenk Gilbert & Sir Arthur Sullivan. (1879). The Pirates of Penzance. https://www.youtube.com/watch?v=uaem0R05xbU
7 Owen-Jackson, C. (2024). The dangers of anthropomorphizing AI: An infosec perspective. Security Intelligence, June 26, 2024. https://securityintelligence.com/articles/anthropomorphizing-ai-danger-infosec-perspective/?mkt_tok=Mjk4LVJTRS02NTAAAAGUQOCU7re403g7pQqpFQiBlVUbQm6exR-V10Cm-ZajQBaMkMXf6KOsYDrEfSiWZm3bDoKe0nr0KWjxDah9gu7ugH8COzVTZn8zLWcO3fIognae6ERjOg