
Decision Intelligence Use Case Examples
By 2027, enterprise intelligence leaders will replace their homegrown decisioning solutions with those built on commercial decision intelligence platforms, accelerating their decision velocity by 2x.1
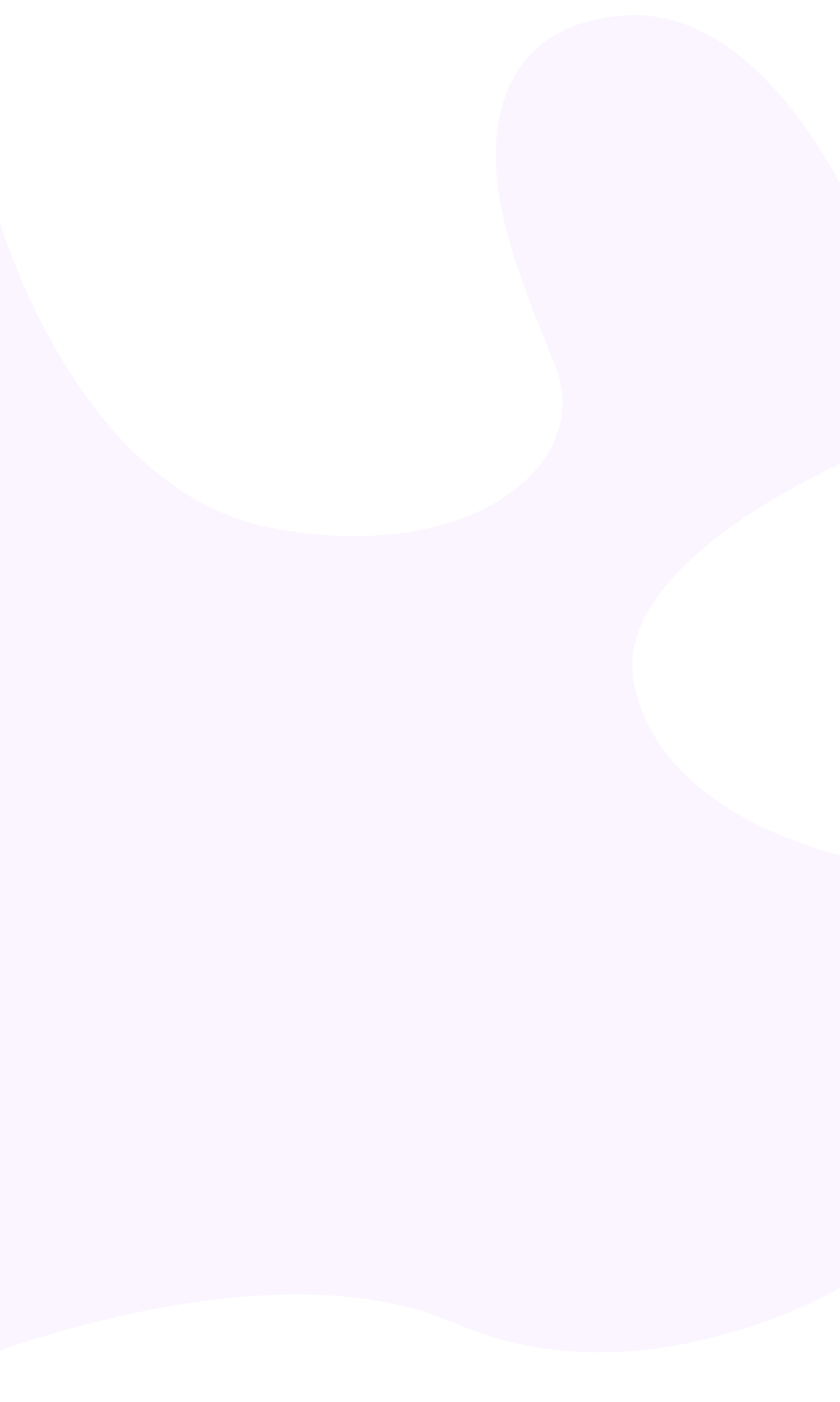
Revealing Social Media Bots
Problem
AI is a force-multiplier and more accessible than ever. Unfortunately, risks associated with AI are as ubiquitous as opportunities. We are now on the cusp of AI bot swarms overwhelming social media platforms (SMP), where YouTube and other providers get nefarious comments submitted by bots to promote fraudulent information or financial scams. Besides impacting provider operations and costs, AI bot comments create a poor user experience, exploit customers, and damage reputations
Solution
Cogility has developed a proof-of-concept solution for detecting AI Bot Swarms on YouTube. Using the Cogynt™ Decision Intelligence Platform (DIP), we have demonstrated an extensible AI Bot Swarm detection model that doesn't require the user to develop any code and that avoids the mass storage of terabytes upon terabytes of comment data. Beyond identifying conspicuous bot comments, Cogynt was able to detect (with irrefutable evidence) inconspicuous bot comments and associated accounts in real-time that human analysts and Google’s sophisticated filters would otherwise have failed to detect.
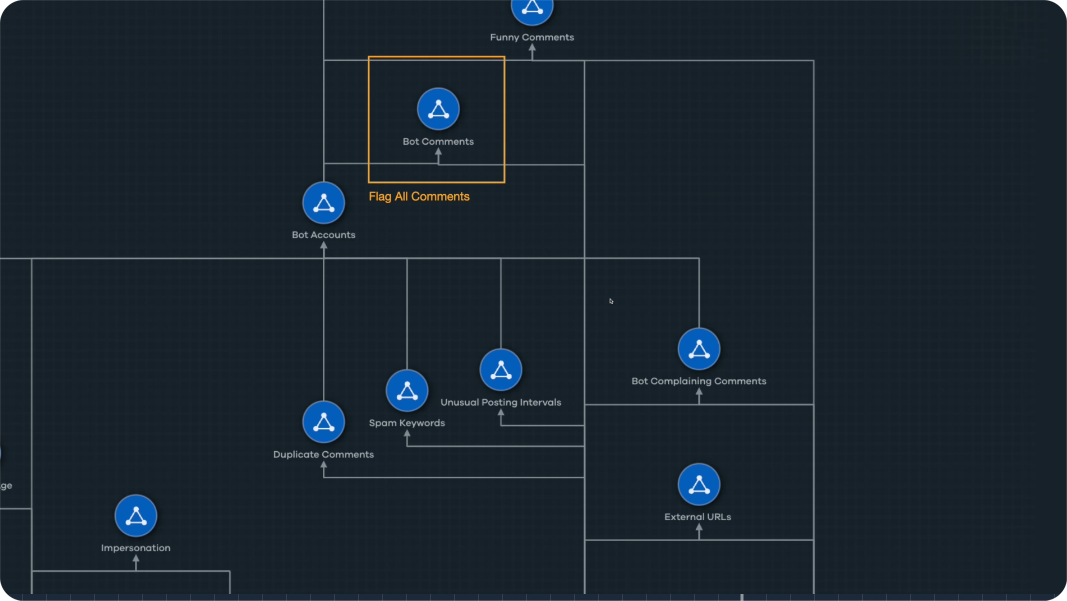
Bank Fraud Detection
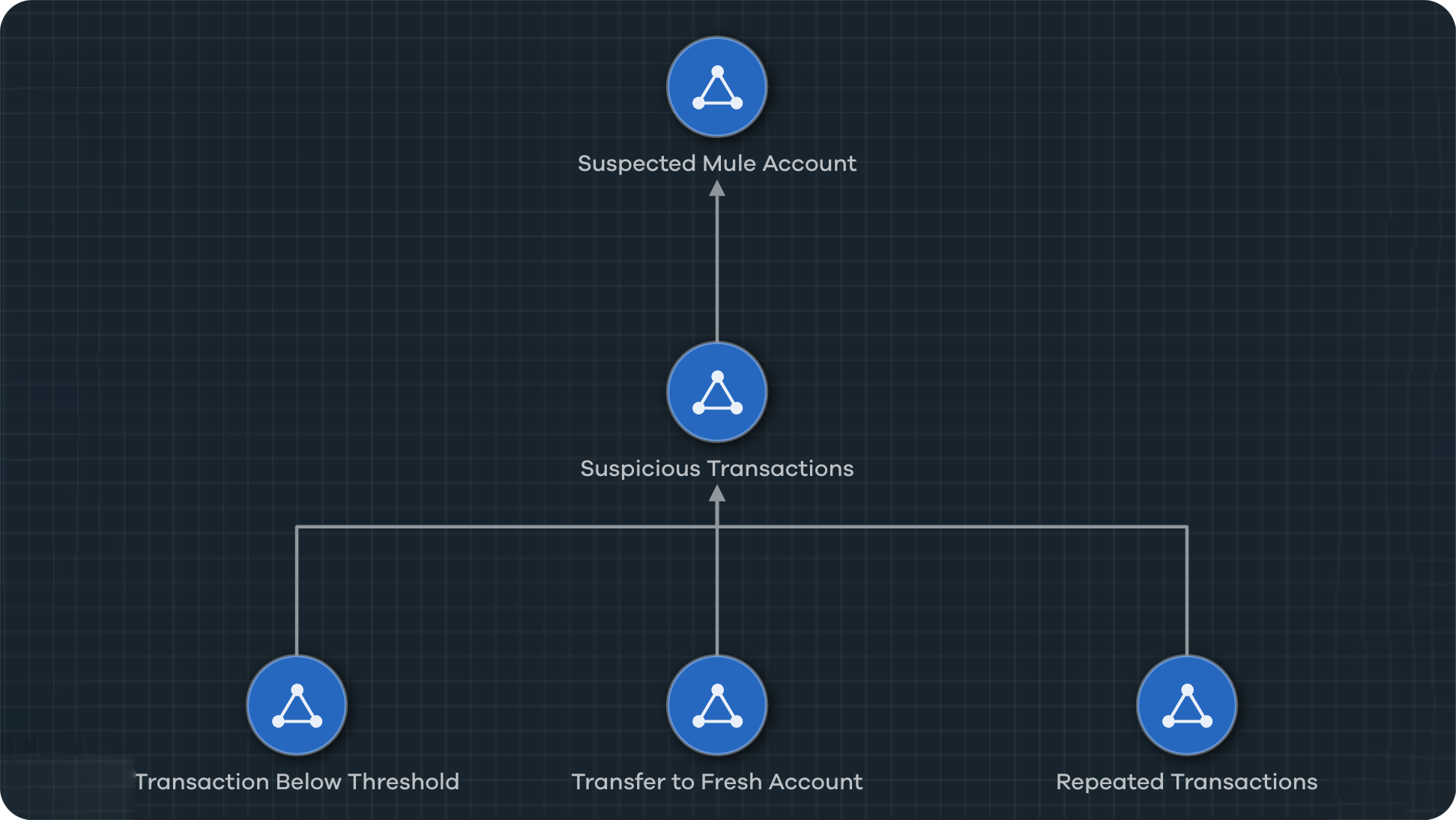
Problem
Bank fraud is a set of schemes that deceive a financial institution to obtain or avoid losing money, property, services, or advantage. While there are many forms of financial fraud, criminal money laundering is an ongoing and pervasive worldwide economic threat. One popular method is the money mule, where illegally acquired money is transferred to another account on someone else’s behalf. It is easy to disguise and difficult to detect.
Solution
A money mule detection and mitigation solution was developed in the Cogynt DIP in an extensible DI model covering new accounts and transaction parameters and analyzing events and patterns of events (short-term and long-term). Emulating a real-world approach to fraud detection, Cogynt was able to identify (with irrefutable evidence) a wide variety of mule account usage in real-time that may otherwise have evaded conventional fraud detection methods.
Causal Forecasting – Ride Share Network
Problem
Managing the logistics within a highly dynamic marketplace is no small task. For example, a ride share volume and profitability can change instantly by any number of variables. Causal modeling is used by data scientists to understand the impact an event or combination of events has on the outcome of other events that could directly affect a company's objectives. Causal modeling is powerful, but typically relies on advanced mathematical and programming skills, such as coding in Python or PyTorch, leveraging statistical models, and more — making causal modeling difficult for organizations to implement.
Solution
The Cogynt DIP for continuous decision intelligence lets any operation tap into the power of causal modeling. Cogynt's model authoring user interface greatly simplifies the process. With Cogynt, analysts and data scientists can not only perform causal forecasting using a drag-and-drop tool, but you can throw a firehose of data at it and see accurate outcome events in real time. Examine how to use Cogynt to assemble a causal forecasting model for operations of any size.
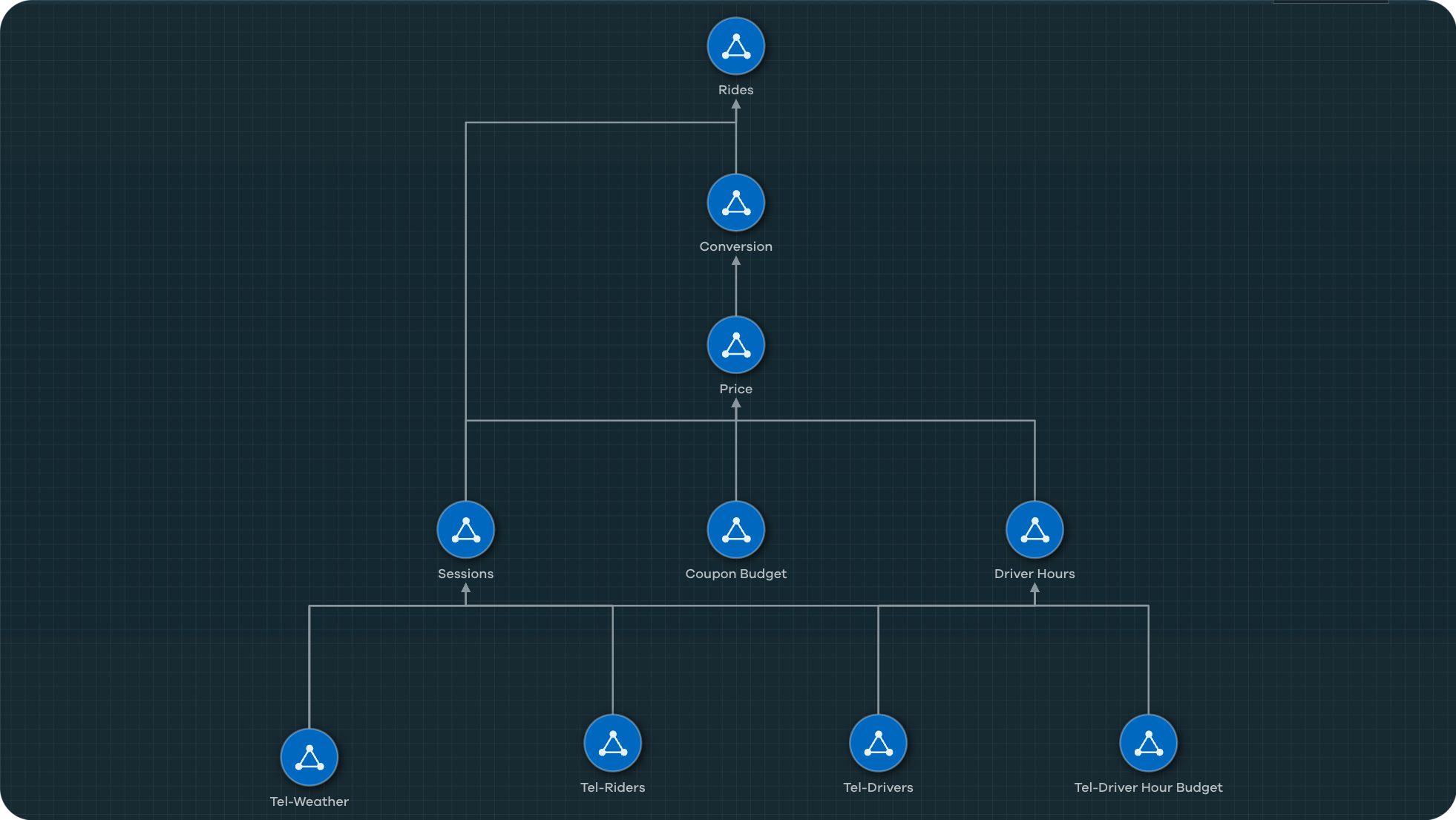
Real-Time Event Processing – Stock Valuation
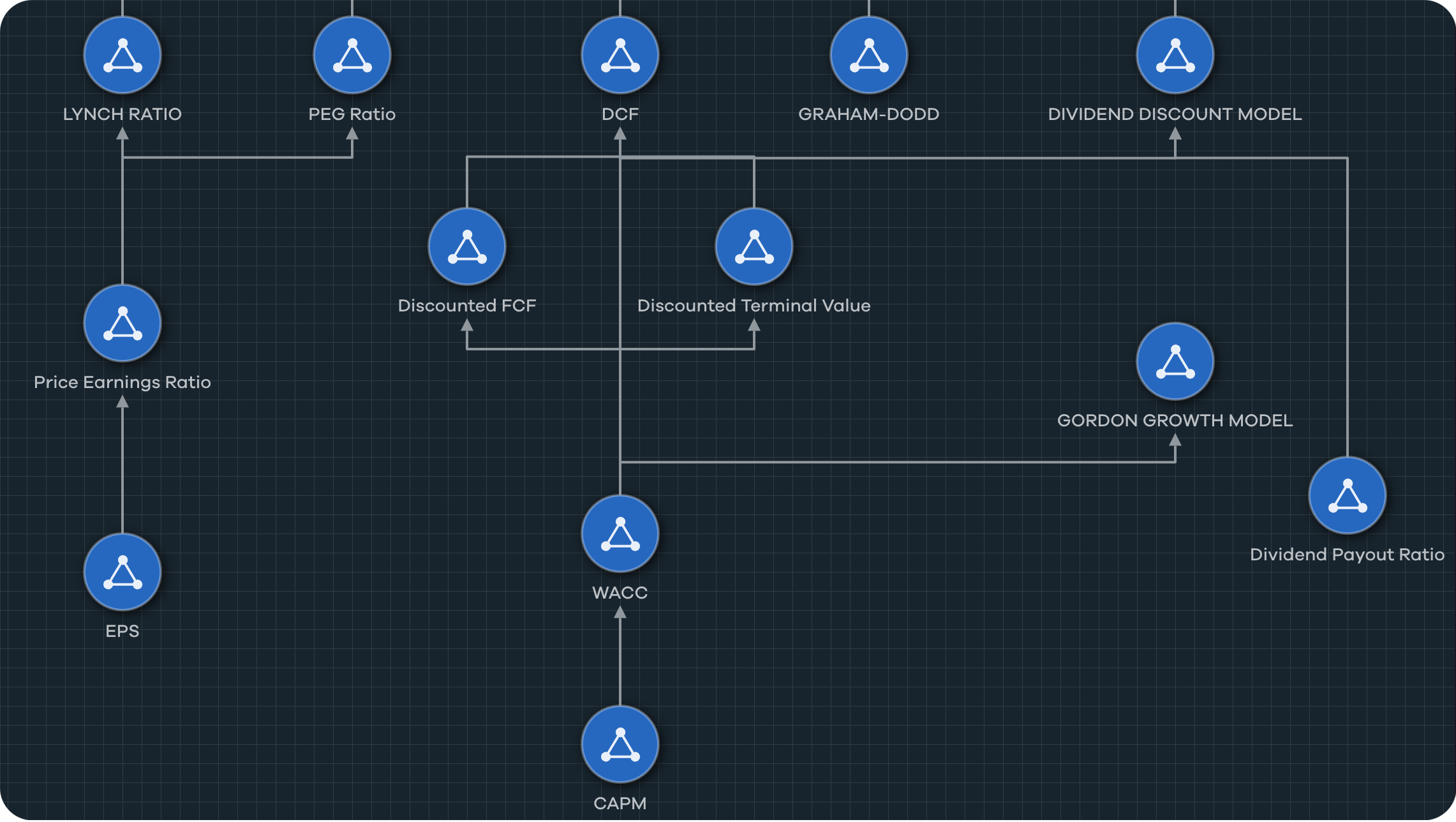
Problem
Understanding the dynamics of financial markets is challenging for any financial services analytics team. What is a stock's intrinsic value? What do a company's financials or other market data (such as shifts in trends or consumer attitudes) tell us about its security and investability? How easy is it to contextualize this information and share the insights to different decision makers? The challenge is how to develop and refine continuous intelligence solutions for the wide array of financial service data analytics and decision support needs with limited analyst and data scientist resources.
Solution
Cogility Cogynt is a force multiplier for financial service providers who want to develop automated decision support solutions for a wide range of financial data analytics services. Learn more about Cogynt’s no-code visual approach to creating mathematic calculations and developing continuous risk-based analysis from real-time data feeds such as stock ticker data.
Continuous Predictive Insights – Fire Risk Protection
Problem
Managing a power grid is an enormous undertaking of critical infrastructure management. Today, fire hazards present a real and present danger. Power company infrastructure is often located in remote areas where conditions on the ground are difficult to assess — where predicting potential fires or rapidly identifying active fires fast can save lives. Worse, after-the-fact outages and loss of power due to fires often complicates evacuation or rescue attempts.
Solution
Accurately predicting which stations must shut down and when is vital for keeping electricity moving continuously through the grid. Complex coded solutions exist for these kinds of events, but Cogynt's intuitive no-code approach offers visual problem solving. Small fluctuations add up to larger threats that humans can miss. Cogynt's answer is a model built around flagging the conditions that lead to fires before they occur. Cogynt's intuitive no-code approach offers visual problem solving to apply simple to complex calculations in real-time data streams to preempt fire issues.
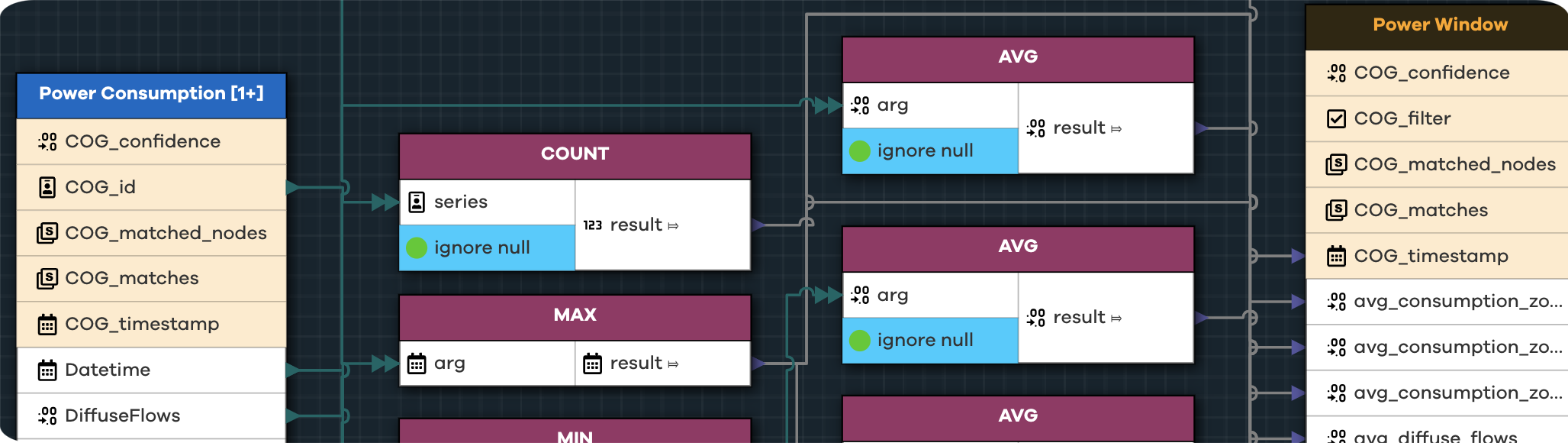
Continuous Intelligence – Fantasy Football
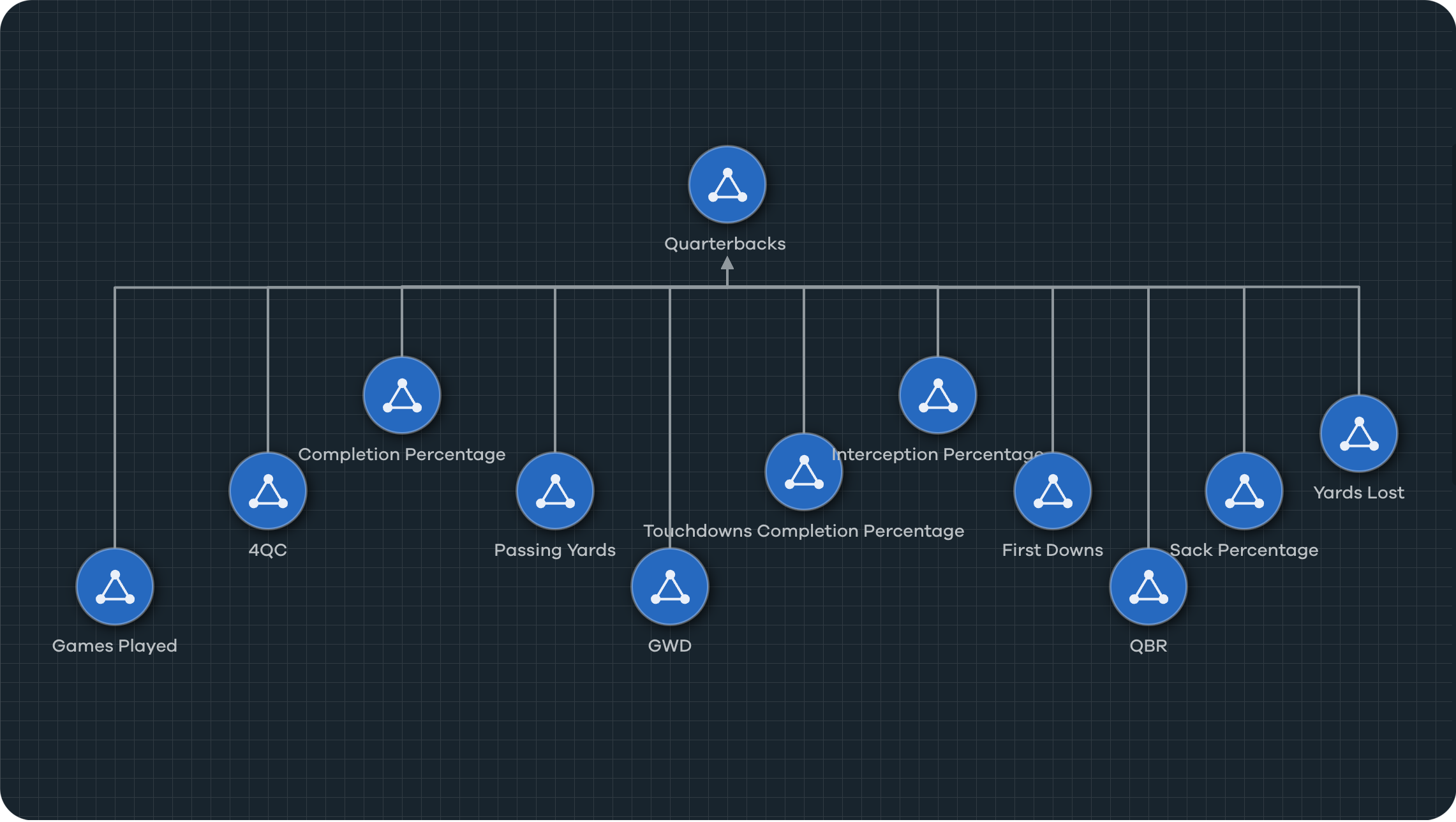
Problem
Which NFL players are poised to have breakout seasons next year? Which of last year's stars are going to regress? Do the league veterans have much more gas left in the tank? How can we build a high performing team. Whether you're competing in a fantasy football league, assembling a competitive high school roster, recruiting for college athletics, or managing a professional club, accurately assessing player performance and potential is key to fielding the best possible team.
Solution
The Cogynt platform is ideally suited to solve this kind of problem. With its ability to accurately calculate risk and manage unknowns, Cogynt can help provide the clearest possible picture from the available data. In this exercise, we use Cogynt to analyze quarterback (QB) statistics throughout a season to gauge their potential upside or downside if signed the following season. The model uses data scraped from Pro Football Reference for the 2019 NFL season. Organizations can apply this methodology to any large, complex volumes of data.
Other Resources
1 IDC FutureScape: Worldwide Future of Enterprise Intelligence 2024 Predictions, 2023